Increasing transferability for automated fault detection and diagnosis in HVAC systems through a hybrid AI methodology” Nicolas Rehault, Fraunhofer Institut for Solar Energy Systems
Wednesday 25 September 2024 | 16:00-17:30 | Briefing Room IT | Paper Session | Hybrid
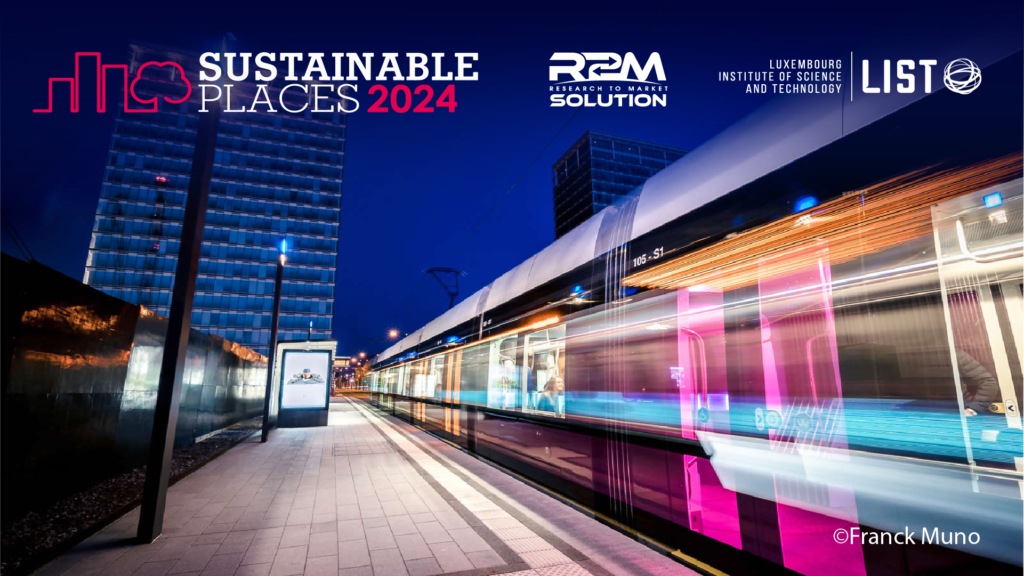
The optimization of the building energy operation with analytic tools like automated fault detection and diagnosis (FDD) can bring significant energy savings and help tackle the global GHG emissions reduction goals. Average energy savings of about 10% using FDD have been demonstrated in several pilot buildings worldwide. Today, most of the FDD solutions available on the market are using rule-based systems that stand out thanks to their simplicity and high specificity. However, rule-based systems require many parameters and significant efforts to be set up, tuned, and maintained. Their transferability from one system to another can consequently be impeded. It is therefore interesting to investigate FDD solutions based on machine learning models, which can perform as well as rule-based systems if well-trained and, furthermore, detect novel fault patterns for which no rule exists. Despite intensive research, solutions based on machine learning models have rarely been implemented in practice for the supervision of Heating, Ventilation, and Air Conditioning (HVAC) systems.
With the aim of achieving a high degree of transferability, we propose a methodology including two different AI methods to model a residual generating FDD approach. The nominal behaviour of the HVAC system is estimated using a bank of multi-layer perceptron networks (MLP) trained on historical operating data and Bayesian optimisation for hyperparameter tuning. For a high-quality estimate, fault-free training data is essential. Therefore, a rule-based system consisting of a large collection of expert rules is used to initially annotate the acquired data. Based on available data points (sensors, actuators, operating signals), the rules are automatically triggered, and for each fault type, a binary signal is generated. This enables an initial detection of known and frequently occurring faults to filter the time series data, which is used for model training. During application, the estimates are compared with the observations. In the event of a fault, the calculated residuals carry information about the fault and can be further evaluated for fault detection and diagnosis. In contrast to a purely rule-based approach, this approach enables the detection of unknown faults.
Applied to several different data sets of ventilation systems from three different buildings, we show that the methodology can be implemented with low parameterisation effort and at the same time outperforms a reference rule-based system in terms of sensitivity and specificity. For fault detection based on the calculated residuals, we introduce a dynamic scoring method depending on the operating mode of the system to increase transferability. The scoring adapts automatically to the quality of estimate and thus prevents frequent false alarms in modes with an insufficient historical data basis and stays sensitive in well-learned modes.
Session Chair:
Nicolas Réhault, Fraunhofer Institut for Solar Energy Systems (ISE) – Sebastian Dietz, University of Luxembourg
Workshop Chair: Sébastien Faye, LIST, Luxembourg Institute of Science and Technology